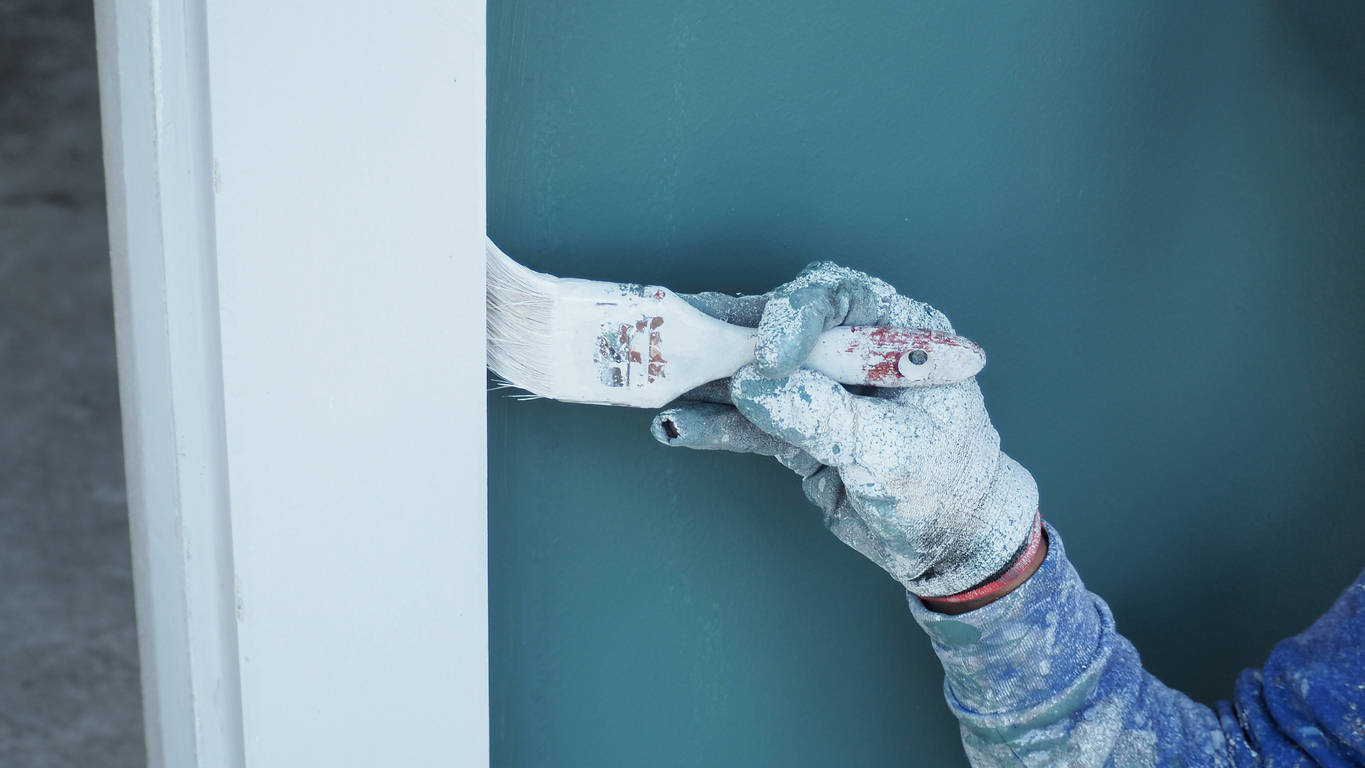
Historically, employers have had discretion in deciding whether to offer group health plan coverage to their employees. For many businesses, this choice no longer exists, and compliant coverage must be offered pursuant to the Patient Protection and Affordable Care Act (the “ACA”) and Section 4980H of the Internal Revenue Code (the “Code”). Despite various challenges to the ACA, these mandates (and the corresponding penalties) are here to stay.
Under these rules, “applicable large employers” or “ALEs” are subject to significant penalties if they (1) fail to offer minimum essential coverage to at least 95 percent of their full-time employees (and their dependents) or (2) offer coverage that is not “affordable” or that does not provide “minimum value.” Employers must report offers of coverage to the Internal Revenue Service and to certain employees on an annual basis. Penalties for noncompliance can be significant.
An employer is considered an ALE if it employed at least fifty full-time and full-time equivalent employees in the preceding calendar year. This low threshold, combined with sophisticated counting rules and limited transition relief, means that small-but-growing businesses are often caught unaware and at risk of penalty.
This article is designed to help employers navigate the complexities of this space and assess their ongoing compliance efforts. Note, however, that the ACA and related guidance offer significantly more detail than what is contained here and should be consulted in all cases. Employers should not rely on this article as legal advice and should consult with legal counsel, as appropriate, based on their own facts and circumstances.
Part I: Determining Applicable Large Employer Status
A. The Rule
An employer must be considered an “applicable large employer” to be subject to a Section 4980H penalty with respect to a given calendar year. An “applicable large employer” is generally one that—in the preceding calendar year—employed at least fifty full-time employees or a combination of full-time and full-time equivalent employees that equals at least fifty. ALE status may change year-to-year and should be evaluated at least annually.
Example. In 2023, Company A employed forty full-time employees (i.e., employees averaging thirty or more hours per week) and ten full-time equivalent employees (e.g., twenty employees averaging fifteen hours per week). Company A is an applicable large employer for calendar year 2024.
B. The Calculation
An employer may follow the steps listed below to help determine its ALE status in a given year. Again, this calculation is for educational purposes only and should not be relied on as determinative on given facts and circumstances.
- Identify the number of full-time employees (i.e., employees working 120 or more hours per month) in each month.
- Add the total number of hours of service for all part-time employees (i.e., employees working less than 120 hours per month) in each month.
- Divide the total hours of service for all part-time employees by 120 to determine the number of full-time equivalent employees for each month.
- Add the number of full-time and full-time equivalent employees in each month. Do not round up or down.
- Average across the year by adding the total number of full-time and full-time equivalent employees and dividing by 12. Round down to the next whole number.
- If the average is 50 or greater, consider whether any special rules apply with respect to seasonal employees or otherwise.
C. Some Nuances
All types of employers can be ALEs. All employers are potentially subject to the employer shared responsibility rules, including for-profit, non-profit, and governmental entities.
Related companies are combined. Employers must count all full-time and full-time equivalent employees across all related employers. If the combined total of all such employees meets or exceeds the ALE threshold, then each related employer is considered an ALE (even if a given company has fewer than fifty full-time and full-time equivalent employees). For this purpose, related employers are determined by reference to the controlled group and affiliated service group rules set out in Code Sections 414(b), (c), (m), and (o).
Counting common-law employees. All common-law employees of the employer must be counted. As a result, careful consideration should be given to the correct classification of independent contractors and temporary employees. Failing to count all common-law employees could cause an employer to conclude incorrectly that it is not an ALE.
Certain individuals are excluded from the count. Sole proprietors, partners in a partnership, 2 percent S-corporation shareholders, leased employees (as defined in Code Section 414(n)(2)), and workers described in Code Section 3508 (i.e., certain real estate agents) are not considered “employees” for this purpose and are excluded from the count. In addition, most employees who work outside the United States are excluded, as are employees who have coverage under TRICARE or a Veterans Affairs’ health program.
Full-time employees. A full-time employee for any month is an employee (including a seasonal worker) who is employed for an average of at least thirty hours of service per week, or 120 hours of service in a calendar month.
Full-time equivalent employees. A full-time equivalent employee for any month is an employee (including a seasonal worker) who is not employed on a full-time basis. To determine the number of full-time equivalent employees in a month, (1) calculate the aggregate hours of service for all employees who are not full-time employees in the month, and (2) divide the total aggregate hours by 120. For this purpose, do not count more than 120 hours of service for any employee.
Hours of service. In general, an “hour of service” includes each hour for which an employee is paid or entitled to pay for the performance of duties and each hour for which the employee is paid or entitled to pay during a period for which no services are performed due to vacation, holiday, illness, incapacity (including disability), layoff, jury duty, military duty, or leave of absence. Certain unpaid leave must also be counted.
Hours for hourly employees. Hours of service include only actual hours with respect to hourly employees. Employers may not apply an equivalency or other estimate of hours.
Hours for salaried employees. Hours of service can consist of actual hours with respect to salaried employees. However, employers may substitute an equivalent number of hours for actual hours, including eight hours of service per day for any day during which the employee was paid or entitled to pay for at least one hour or forty hours of service per week for any week during which the employee was paid or entitled to pay for at least one hour. These equivalency methods must be applied uniformly across reasonable classifications of employees. Employers may not use an equivalency if it would result in a substantial underreporting of hours.
Seasonal worker exclusion. If the total number of full-time employees and full-time equivalent employees is fifty or greater for 120 or fewer days, or four or fewer calendar months, in a calendar year, and the employees in excess of fifty employed in that period were seasonal workers, the employer is not an ALE. The 120 days and the four calendar months are not required to be consecutive. For this purpose, employers may apply a reasonable, good faith interpretation of the term “seasonal worker.”
Part II: Calculating Penalties
A. The Rule
Large employers are subject to two potential coverage-related penalties: the Section 4980H(a) penalty and the Section 4980H(b) penalty. The former is often the larger and more significant of the two, as it is determined as a multiple of all full-time employees. ALEs may also be subject to significant reporting penalties. All penalties are determined monthly and are enforced by the Internal Revenue Service (IRS) on a rolling basis.
B. The Calculations
Section 4980H(a) penalty. A Section 4980H(a) penalty is due for any month in which (1) an employer does not offer “minimum essential coverage” to at least 95 percent of its full-time employees and their dependents and (2) at least one full-time employee receives a premium tax credit to help pay for coverage on a health insurance marketplace. This penalty applies with respect to all but thirty full-time employees, not just those who receive premium tax credits or those who were not offered coverage. The Section 4980H(a) penalty is paid by the employer. The annual Section 4980H(a) penalty for 2024 is $2,970 per employee to whom the penalty applies.
Section 4980H(b) penalty. A Section 4980H(b) penalty is due for any month in which (1) the coverage offered by the employer is unaffordable or does not provide minimum value and (2) at least one full-time employee receives a premium tax credit to help pay for coverage on a health insurance marketplace. Unlike the Section 4980H(a) penalty, the Section 4980H(b) penalty applies only with respect to employees who receive a premium tax credit. The Section 4980H(b) penalty is paid by the employer. The annual Section 4980H(b) penalty for 2023 is $4,460 per employee to whom the penalty applies.
Reporting penalties. A reporting penalty is due for any year in which the employer failed (1) to file complete and accurate information returns with the IRS or (2) to furnish complete and accurate information returns to applicable full-time employees. These penalties are determined by reference to the number of delinquent or inaccurate forms filed or furnished. The reporting penalties are paid by the employer. The penalty for 2024 is $310 (for failure to file) and $310 (for failure to furnish) per form.
C. Some Nuances
Penalties adjusted for inflation. Each of the penalties is adjusted annually for inflation.
Coverage penalties are alternatives. The IRS may assess only the greater of the Section 4980H(a) and Section 4980H(b) penalties in any given month. However, coverage penalties and reporting penalties may be assessed in tandem.
Related companies are not combined. Although the related business rules apply for determining whether an employer is an ALE, the penalty calculation applies on a company-by-company basis. Each company within a controlled group or affiliated service group separately files information returns and assesses penalty exposure.
Allocation of thirty-employee reduction. The Section 4980H(a) penalty permits a thirty-employee offset, which is allocated pro rata among an employer’s related companies. If a company’s allocation is fractional, round up to 1. The regulations do not permit employers to allocate this reduction at will (i.e., it must be spread ratably).
Offers of coverage. Employees must be provided an effective opportunity to accept or decline health insurance in order to be treated as having been offered coverage. An offer of coverage must be made to employees at least once each plan year.
Related company offers. An offer of coverage by one company to an employee for a calendar month is treated as an offer of coverage by all related companies for that calendar month.
The 95 percent test. The regulations provide that an ALE will be treated as offering coverage to substantially all its full-time employees and their dependents for a calendar month if it offers coverage to all but 5 percent, or if greater, five, of its full-time employees in that month.
Margin of error. Technically, employers have a 5 percent margin of error with respect to Section 4980H(a) compliance. Nevertheless, a penalty may still be assessed even for small, inadvertent errors (e.g., offers to 94.9 percent of full-time employees and their dependents).
Dependents. For this purpose, an employee is treated as having been offered coverage only if the employer also offers coverage to the employee’s dependents. A “dependent” means a natural child or an adopted child until the end of the month in which the child attains age twenty-six. Spouses, stepchildren, and foster children are not considered dependents and do not need to be offered coverage.
Minimum essential coverage. Minimum essential coverage is defined to include any group health plan or group health insurance that is not a Health Insurance Portability and Accountability Act (HIPAA) excepted benefit (e.g., standalone dental or vision plans).
Minimum value. A plan provides minimum value if it covers at least 60 percent of the total allowed cost of benefits that are expected to be incurred under the Plan. The Department of Health and Human Services developed a minimum value calculator to help determine if a plan with standard features provides minimum value. Plans with nonstandard features are required to obtain an actuarial certification. The regulations also describe certain safe harbor plan designs that satisfy the minimum value standard.
Affordability. Coverage is considered “affordable” if the lowest-cost self-only premium is less than 9.5 percent (as adjusted annually) of the employee’s annual household income. Given that household income is difficult to ascertain, the regulations include three safe harbors that may be relied upon to determine affordability. Use of the safe harbors is optional, and an employer may elect to apply them to any reasonable category of employees on a uniform and consistent basis. For this purpose, a reasonable classification can include specified job categories, nature of compensation, geographic location, and other bona fide business criteria.
Full-time employee count. The coverage penalties hinge on whether an ALE offers affordable, minimum essential coverage that provides minimum value to its full-time employees and their dependents. The IRS permits only two methods for determining whether an employee is “full time” for this purpose: the monthly measurement method and the look-back measurement method, which are beyond the scope of this article. These methods are different than the approach described in Part I with respect to determining ALE status.
Part III: Penalty Mitigation Strategies
Monitor ALE status. An employer’s status as an ALE may change from year-to-year, such that it is important (especially for small-but-growing businesses) to actively monitor employee count.
Section 4980H(a) penalty mitigation. To lower the risk of being assessed a penalty under Section 4980H(a), an employer should offer minimum essential coverage to at least 95 percent of its full-time employees and their dependents in each month of the year.
- Cost considerations. Certain employers elect to pay the penalty rather than bear the cost of insurance. Keep in mind that penalties are not deductible, unlike health insurance costs. Note also that employers may require employees to pay the full cost of coverage at the risk of incurring potentially smaller Section 4980H(b) penalties.
- Plan documents. Confirm that the group health plan documents provide for a thirty-hours-of-service requirement (or lower) and cover employees and dependents to age twenty-six. Some employers may decide to apply a more liberal eligibility definition (e.g., a twenty-hour standard) to provide a margin of error.
- Eligibility alignment. Consider using the look-back measurement method for both reporting and eligibility and adopting a corresponding policy.
- Margin of error. Monitor hours and employment status to preserve the 5 percent margin of error under the 95 percent test.
Section 4980H(b) penalty mitigation. To mitigate the risk of being assessed a Section 4980H(b) penalty, an employer should confirm that coverage provides minimum value and that the lowest-cost self-only premium satisfies the applicable affordability safe harbor for as many full-time employees as possible.
Reporting penalty mitigation. To mitigate the risk of being assessed reporting penalties, an employer should establish strong procedures for accurately and timely filing and furnishing Forms 1094-C and 1095-C. Many employers hire third-party vendors to complete reporting, but these relationships should be closely curated and monitored. Forms (and their underlying data) should be reviewed before filing, with special attention paid to the “offer of coverage indicator” and “full-time employee count” on Form 1094-C.
Matthew is grateful for the support and contributions of his partner, Nancy Campbell, in the preparation of this article.